Increasing The Probability Of Success - Part 2
In part two of this series, we continue the discussion of different approaches to using probabilities in probability-weighted returns.
2. PROBABILITY BUCKETS
In many ways, this is the easiest of all the methods to implement. Predetermine as a firm how many price target scenarios you’re going to forecast per position. For example, let’s say you’re going to do three: Reward, Base, Risk. And for each position, the analyst can choose Low, Medium, or High Probability and you preset the probability distribution. For instance:
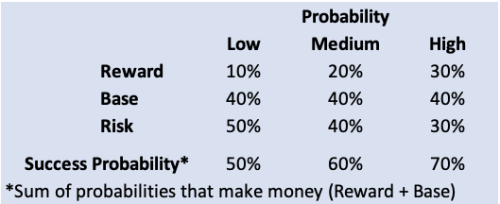
In this case, probabilities are constrained within a range but allow for some flexibility. What you should expect from your analysts is a normal distribution of probability ranges. Mostly Mediums with a few Lows and Highs. No analyst should have more Highs than anything else. The way to explain this to your team is that Low isn’t bad. It’s just less likely than the average (Medium) name. And vice versa for a High.
Probability Buckets are the most common probability recommendation we’ve made for clients. They are a good combination of flexibility and practicability, easy to explain to the team, and an effective audit to determine if analysts are being too aggressive.
3. FLEXIBLE PROBABILITIES
The book Superforecasting explains how individuals can improve their forecasting skill (if you are a forecasting practitioner you should read the book – see our blog post about it here). One primary trait of Superforecasters is micro-updates. Superforecasters change their probability estimates in small increments with new information. For example, changing their probability of Trump winning the 2016 election from 43% to 45% after getting the latest polling data. These small updates accrue to better forecasting accuracy.
Given that fact, you’d think my recommendation would be for Alpha Theory clients to make micro-updates and have lots of flexibility with price targets. My answer is, well, it depends. The incentive for Superforecasters was to maximize their accuracy. The incentive for most analysts is P&L, not accuracy, making them reward seekers. This encourages behavior that increases position size (ie. inflated price targets and probabilities – case in point, the average hit rate for all Alpha Theory clients is 51% however analyst assume they're going to make money 72% of the time).
The culture and workflow of the firm determine what makes the most sense. If analysts come up with price targets and probabilities with little to no input from the Portfolio Manager then use Fixed Probabilities and Probability Buckets to reduce the likelihood of them “gaming the system.” If the opposite is true and price targets and probabilities are a collaborative exercise with the Portfolio Manager, then Flexible Probabilities is more than likely ideal.
One last suggestion as it relates to setting probabilities: know your funds' historical hit rate. What percentage of positions made money on an absolute basis? And on an alpha basis? What percentage hit their upside price target? What percentage hit their downside price target? Use these to set a baseline for the probabilities of the firm. For example, if the historical batting average of the firm is 51%, then the average probability of hitting the reward target should not be 72%. Keep your probabilities realistic and the portfolio you build will be a more accurate forecast of what you will receive (see the “Probability Inflation” blog post).
There is no question that setting probabilities is one of the trickiest parts of scenario-based forecasting. We hope this overview gives you a starting point for how to implement an effective probability setting framework.