Noise: The Maker Of Markets And Madness
Where there is noise, there is room for improvement. In this article, Cameron Hight elaborates on how to reduce the noise in your investment process and increase returns.
This article is written in collaboration with Warren Hatch, CFA (Good Judgment Inc.).
Reflect on your own investment process and ask this question: “Given identical sets of information, would two different analysts come to the same conclusion? Would an analyst come to the same conclusion on different days?” If not, there is noise. Where there is noise, there is room for improvement.
As investors, we accept that market participants have different opinions about the value of securities. That’s what makes a market. Even within investment teams, a diversity of views is a good thing.
The problem is how we combine those views. All too often, different people will use the same process and information to come up with the same (often wrong) answer. You can even ask the same person the same question at different times and get wildly different answers. All this noise is a bad thing. It adds volatility to the data, wastes our time by drawing attention away from the underlying drivers of value, and impairs the quality of our investment decisions.
One option is to ignore the noise, take an average of everyone’s different views, and call it a consensus. That’s what we do with consensus earnings estimates. Or GDP forecasts. Or Wall Street dinner surveys. The wisdom of the crowd works, and over time this is certainly better than relying on any one individual.
A better option is to reduce the noise, not just ignore it. New and exciting research findings by Nobel laureate Daniel Kahneman and others show us concrete steps we can take to identify and reduce noise.
Kahneman often describes noise by citing work he performed for a large insurance company. In the example, two different insurance adjusters were given the same insurance claim and came up with the two substantially different payouts. The difference in those claims is noise. The question becomes, what level of subjectivity should there be in an insurance claim?
The problem is that humans are unreliable decision makers; their judgments are strongly influenced by irrelevant factors, such as their current mood, the time since their last meal, and the weather. We call the chance variability of judgments noise. It is an invisible tax on the bottom line of many companies.
A firm whose employees exercise judgment does not expect decisions to be entirely free of noise. But often noise is far above the level that executives would consider tolerable—and they are completely unaware of it.
Obviously, investors are different than insurance adjusters. They don’t get an insurance claim form with all relevant information. But noise has been studied and repeatedly demonstrated to impact decision making across fields. Kahneman further distinguishes noise from cognitive bias:
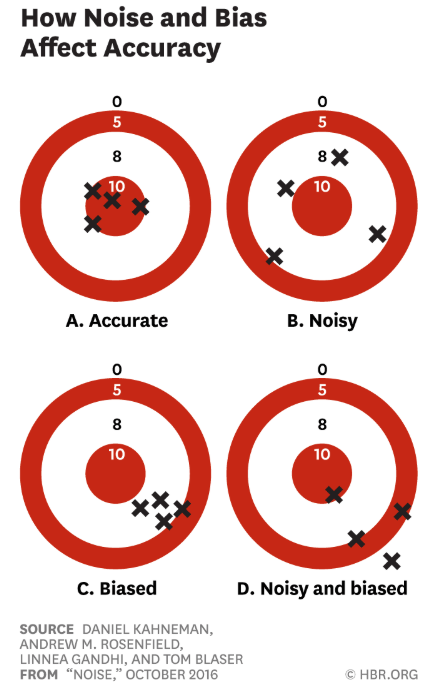
To appreciate the distinction, think of your bathroom scale. We would say that the scale is biased if its readings are generally either too high or too low. If your weight appears to depend on where you happen to place your feet, the scale is noisy. A scale that consistently underestimates true weight by exactly four pounds is seriously biased but free of noise. A scale that gives two different readings when you step on it twice is noisy. Many errors of measurement arise from a combination of bias and noise. Most inexpensive bathroom scales are somewhat biased and quite noisy.
– “Noise: How to Overcome the High, Hidden Costs of Inconsistent Decision Making” – Kahneman, Rosenfield, Gandhi, Blaser (HBR October 2016)\
So how should an investor reduce noise? By increasing process. Kahneman’s “radical solution” is to go all the way and replace human judgment with algorithms. These models can be straightforward: select 6–8 key variables, assign equal weights, and devise the simplest possible rule to combine them. Where replacing human judgment completely with algorithms isn’t viable or even desirable, there are other solutions.
Alpha Theory’s work with clients shows how this process can work. In a matter of 30 minutes, a “simple model” can be created to generally codify the 5-10 most important attributes of a firm’s process. Backed up by experience and empirical results, these simple models can reduce noise and increase returns—and the improvement is immediate and lasting.
Good Judgment Inc, the commercial company built on the research findings by Professor Philip Tetlock and “Superforecasting,” helps clients boost forecast accuracy with a suite of tools to cut through the noise. At recent Superforecasting workshops, for instance, group accuracy improved by an average of at least 20% after just 15 minutes of structured discussion.
Noise is the enemy of good decision making. The separate but complementary work at Good Judgment Inc and Alpha Theory show there are steps you can take now that can reduce noise and boost your bottom line.