Optimizing Usage For Optimal Returns (Part 2) - Position Level Analysis
A follow up to part one, Alpha Theory explores the relationship between forecast freshness and portfolio coverage on one-day forward returns at the ticker level.
In our last edition, “Optimizing Usage for Optimal Returns”, we explored the relationship between forecast freshness and portfolio coverage on one-day forward returns at the fund level. Freshness and performance showed a nearly monotonic relationship. Coverage, on the other hand, had a more parabolic shape with the lowest performance coming from the middle of the range. To explore these relationships further in this edition, we are investigating data at the ticker level instead of the fund level. We ask:
1. Does coverage show more predictive power at the ticker level than it did at the fund level?
2. Is freshness correlated with a performance at the ticker level?
Coverage
Ten years of data on Alpha theory clients have shown that process-oriented investing yields higher returns. A large part of the process centers around entering and updating scenarios forecasting future stock prices, and the probability of them reaching that price. Implicit in this philosophy is the idea that if you are going to make an investment, it should be supported by research. As part of our exploration of coverage and freshness at the ticker level, we regressed coverage against a one-day forward price change. In this case, coverage is treated as a binary variable based on whether a forecast has been made on the position or not.
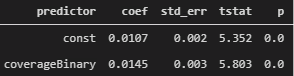
Unlike the results of measuring coverage by the fund, when measured at the ticker level, there is a distinct positive relationship with one-day forward price change. Simply stated, positions with price targets are more likely to outperform positions without price targets.
Freshness
There is more than one way to bake a Christmas cookie, so we first examined which measure of freshness has a stronger relationship with one-day forward price change. We examined two variations. The first measures freshness in terms of the number of days since a forecast was last updated (DSLU). The second treats it as a binary feature, where its value is 1 if price targets were updated in the last ninety days, and zero otherwise. We regressed both features against a one-day forward price change for all positions in Alpha Theory’s historical database. The results for the DSLU method can be found in figure 1, and the results for the binary method in figure 2.
Figure 1 (Days Since Last Update)
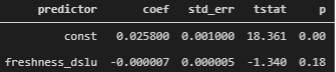
Figure 2 (Binary: Updated in last 90 days)
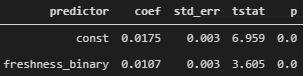
The number of days since the last update has a negative coefficient and t-statistic, which makes sense, given that one might reasonably expect a lower degree of certainty of positive future returns when forecasts are out of date. While intuitive, its lower t-statistic and higher p-value means this relationship deserves further investigation before drawing any conclusions. The binary feature, however, has more conclusive results. Having coverage no older than ninety days does have a positive relationship with the one-day forward price change, with a higher t-statistic and lower p-value.
The importance of creating price targets and keeping coverage fresh can be summarized by the annualized price change of fresh positions vs. stale and uncovered positions.
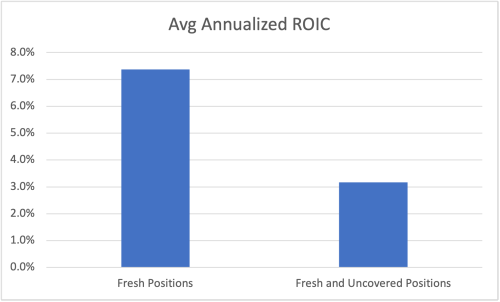
Scattered, Fresh, and Covered
Based on the analysis above, it is well worth the time to create price targets and keep them fresh as they are both important predictors of future returns. When thinking through how your firm is going to improve in 2021, use these empirical proofs as evidence that the team should seek process as a proven way to improve returns.